In this blog, we will explore a use case for AI agents, highlighting how these technologies can be applied in various business scenarios. We’ll also examine the key differences between AI agents and RPA bots, emphasizing their distinct strengths and areas of application. Before diving into the use case, let’s start with a quick overview of these two technologies.
AI Agent Overview
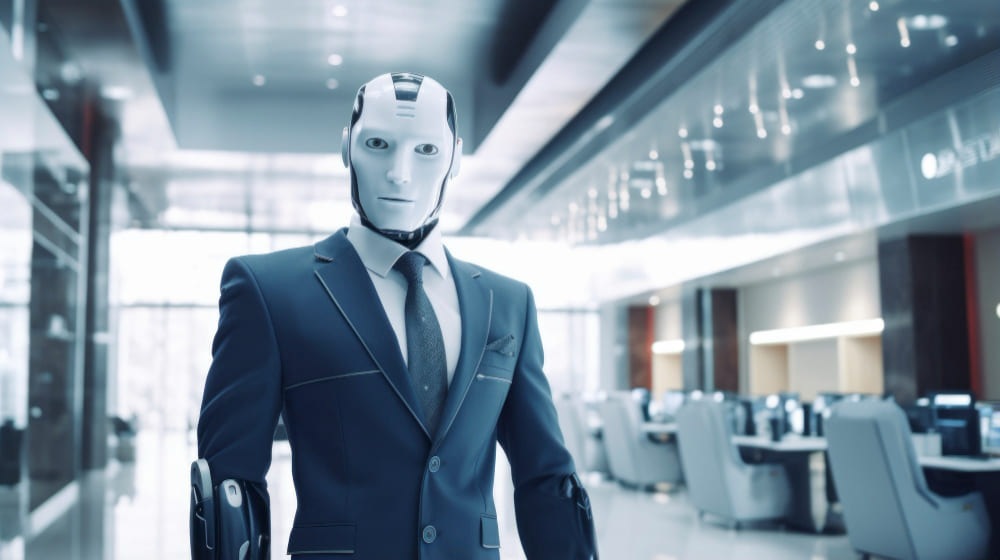
AI Agents use machine learning, NLP, and computer vision to handle complex, unstructured tasks. They can learn, adapt, and make context-based decisions.
Key Traits of AI Agents
- Suited for complex tasks requiring context understanding.
- Adaptive and learns over time.
- High autonomy and independent decision-making.
RPA Overview
RPA (Robotic Process Automation) automates rule-based, repetitive tasks like data entry or invoice processing using software bots. These bots are non-adaptive and follow set rules without learning or decision-making abilities.
Key Traits of RPA
- Ideal for structured, predictable tasks.
- Operates with set instructions.
- Requires reprogramming for changes.
- Limited decision-making and autonomy.
Use Case: Hyper-Personalized Marketing with AI Agents
Overview
AI agents harness extensive customer data from various sources—such as social media interactions, transaction history, and browsing patterns—to deliver hyper-personalized marketing experiences. By analysing this data, they create tailored recommendations, offers, and content that resonate with individual customers across multiple industries.
Examples
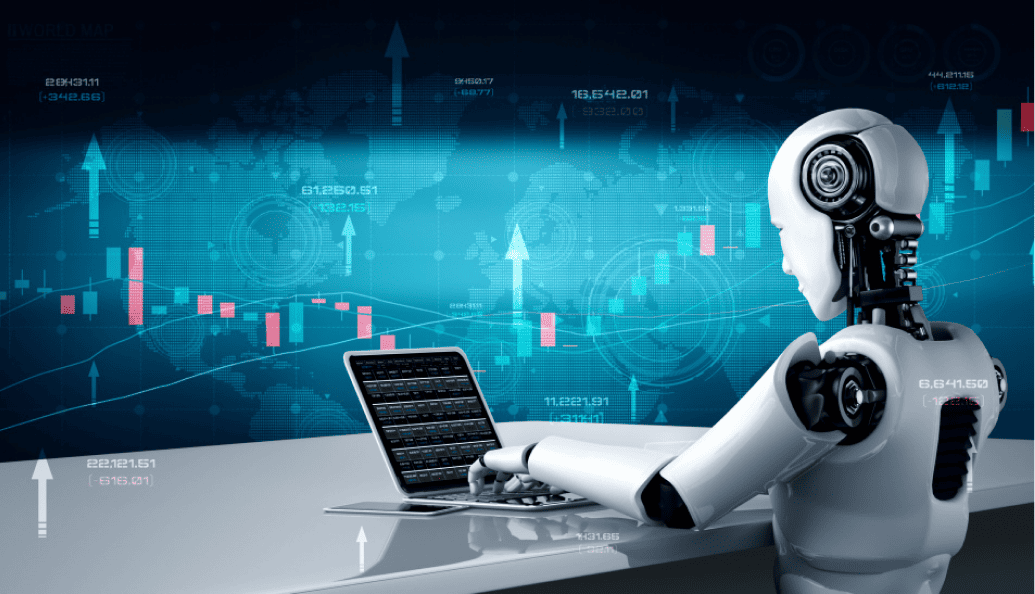
Finance: AI agents can analyse a customer’s spending habits and financial goals to offer personalized investment advice or customized credit card options. For instance, a customer frequently traveling for business might receive offers for travel rewards credit cards that align with their spending patterns.
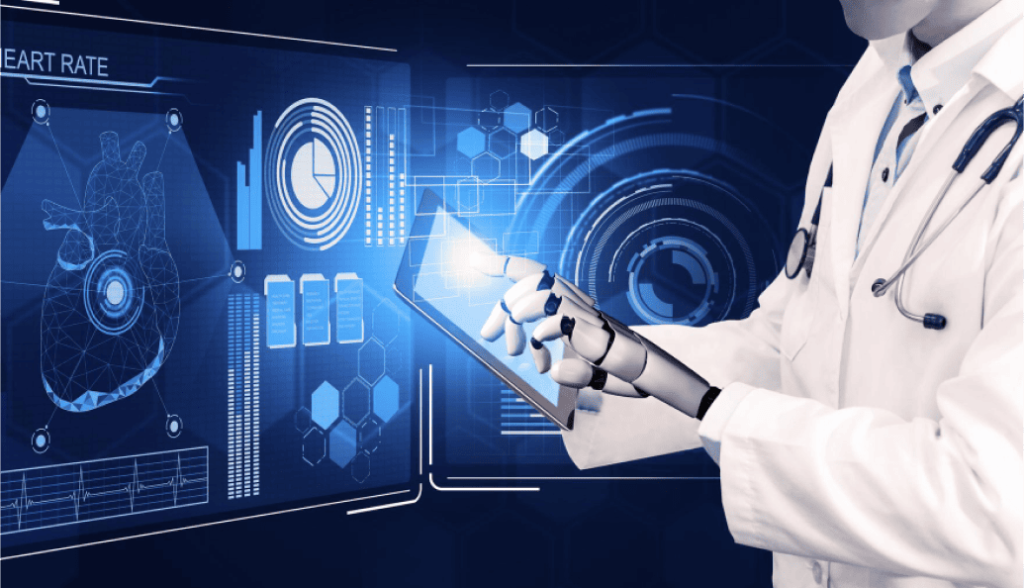
Healthcare: In the healthcare sector, AI agents can develop personalized wellness programs based on a patient’s medical history and real-time health data. For example, an AI agent might suggest specific dietary changes, exercise routines, or regular check-ups tailored to a patient’s health needs.
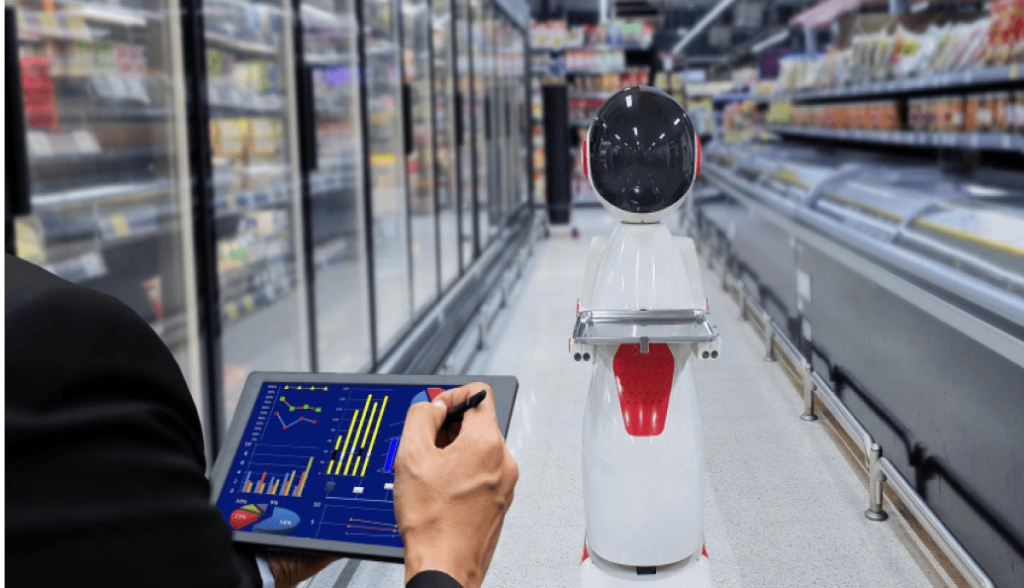
Retail: Retailers, both online and in-store, can utilize AI agents to provide personalized discounts and product recommendations. For instance, a customer who frequently buys sports gear may receive targeted promotions for new athletic products via app notifications or email.
Media and Entertainment: Streaming services can leverage AI agents to curate content recommendations based on user preferences and viewing history. For example, if a user often watches documentaries, the AI agent might suggest similar content or notify them of upcoming releases in that genre.
Value
Enhanced Engagement: Tailored content and offers are more likely to capture customer interest, increasing interaction and satisfaction.
Improved Customer Retention: By providing relevant recommendations and personalized experiences, businesses can build stronger relationships, fostering customer loyalty.
Optimized Resource Allocation: By focusing on highly targeted campaigns, organizations can reduce marketing costs and improve the return on investment.
Scalability: This hyper-personalized approach is adaptable across various industries, making it a versatile strategy for reaching diverse customer bases.
This use case highlights how AI agents can transform marketing strategies by delivering personalized experiences that resonate with customers, ultimately driving engagement and loyalty.
Conclusion
RPA and AI Agents play different roles in automation. Choosing the right tool, or combining both, can enhance efficiency and decision-making in businesses.